
How Deepfakes Work
Understanding the technology

Department of Homeland Security, Increasing Threat of DeepFake Identities
General Adversarial Networks (GANs)
-
The generator takes data inputs and uses pattern learning to generate a video/image.
-
The discriminator is another network that verifies the authenticity of the sample by cross-referencing the sample with a large data pool of real images
-
If the sample is detected to be a deepfake, then the model is reworked and the image is generated again to be sent to the discriminator
-
Steps 2-3 cycle over and over again until the discriminator makes a mistake and is unable to distinguish between the real and fake images
Why are GANs Important?
GANs are the foundations of deepfakes as a whole. It creates the framework that allows these technologies to produce such accurate replications.
​
Deepfakes allow concurrent face-to-lip synchronization, allowing the user to generate realistic videos and images of events that never actually happened. Without the GANs system, this would not be achieveable.

via Voicebot.ai
The gif above shows an example of how existing data on the internet can be altered. For instance, on the top, AI was fed clips of Brad Pitt, which was then used to replicate the features onto the Mona Lisa.
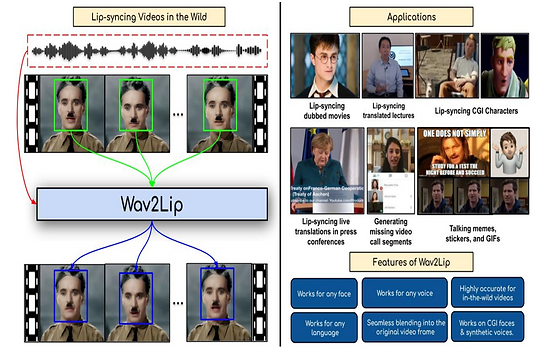
Department of Homeland Security, Increasing Threat of DeepFake Identities
The illustrations above demonstrate how Wav2Lip operates.
​
Wav2Lip provides services that allow users to synchronize lip-sync target speech to any video. Platforms such as these enhance the quality of deepfakes by producing alarmingly accurate speech-to-audio transitions.
​
Thus, as shown in the gif above, the produced videos would undergo video and audio encodings before it pieces together the frames to produce the final result.